Solutions to Help Community Financial Institutions Compete
Contact Us →Houston,TX SBA Trading and Sales Team Joins SouthState|Duncan Williams
View Press Release →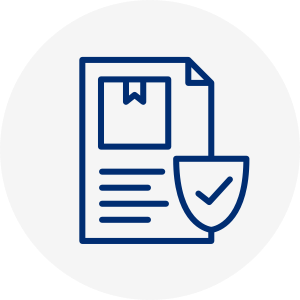
ARC Loan Hedging
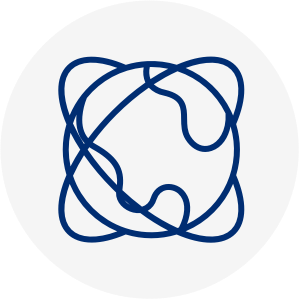
International Services
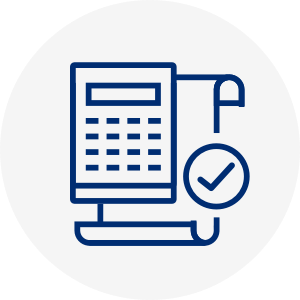
Debt Capital Markets
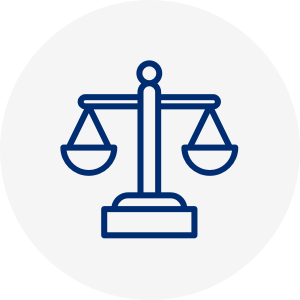
Clearing & Cash Management
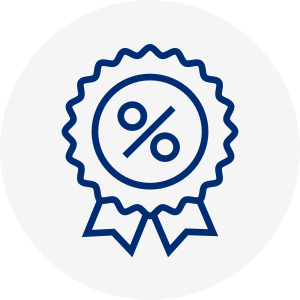
Loan Pricing Model
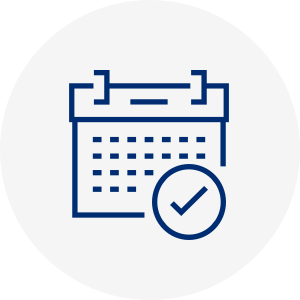
Upcoming Events
Banker-To-Banker Blog
The Banker-To-Banker blog details the latest data, strategies, and tactics that can save your bank resources and provide performance-enhancing ideas to keep you ahead of the competition.
READ THE BLOGMust-Read Insights on the Economy, Regulation, and Bank Leadership
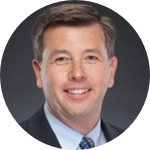
Market Insights & Commentary
Tom FitzgeraldDirector of Research & Strategy
Market commentary and research articles on the overall economy as well as opportunities and risks in the fixed income markets with a focus on helping your bank meet its earnings objectives.
READ MARKET INSIGHTS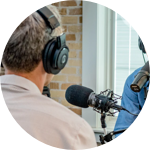
Community Bank Podcast
SouthStateCorrespondent Division
The podcast dedicated to helping community bankers grow themselves, their team, and their profits.
LISTEN NOW